Jiatong Li
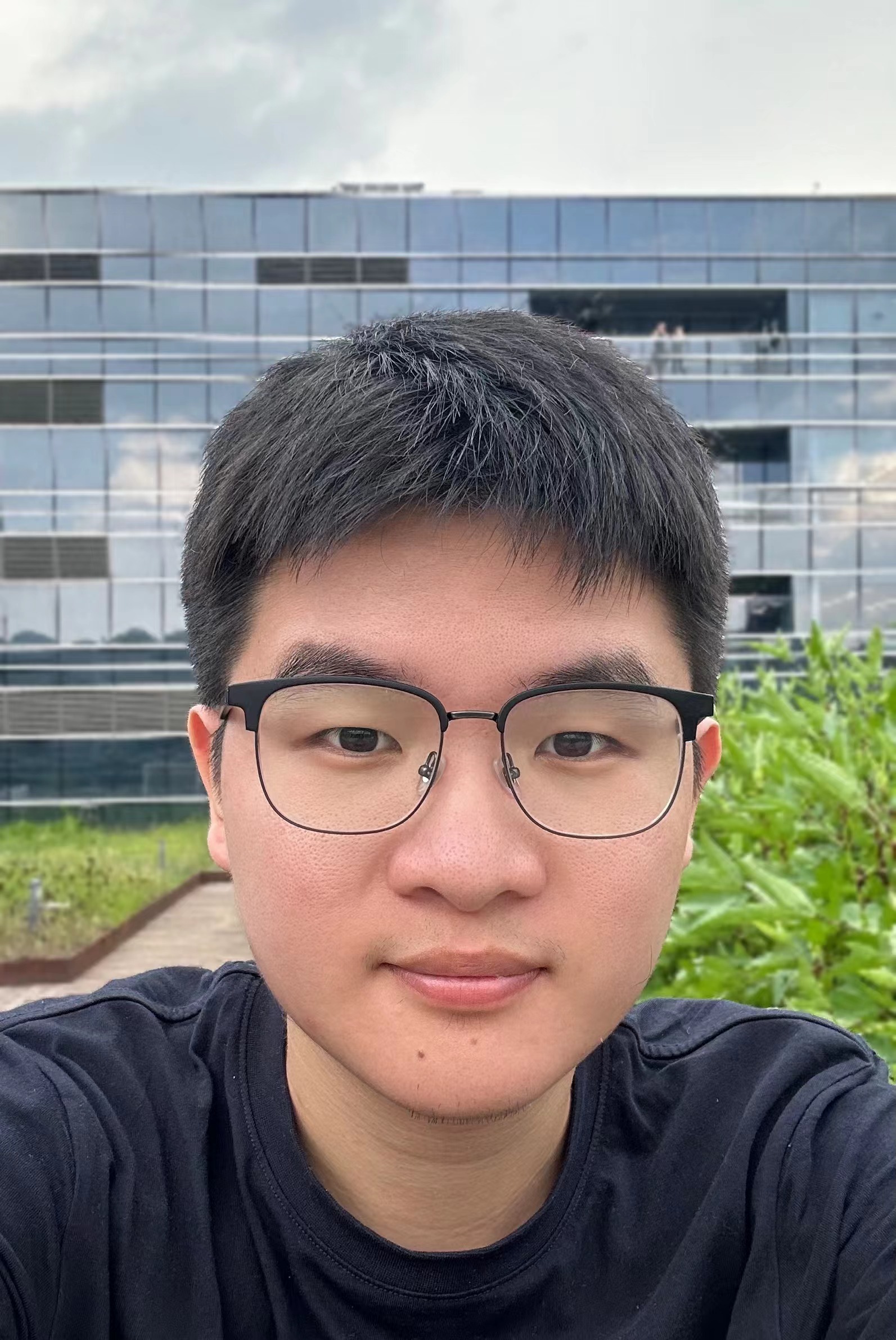
cslijt[at]mail.ustc.edu.cn
I am a master’s student major in data science at University of Science and Technology of China (USTC), advised by Prof. Qi Liu. I received my bachelor’s degree in data science at USTC in 2022. My research interest includes trustworthy ML, LLM, and educational data mining.
Previously, I was fortunated to work with Dr. Mengxiao Zhu at USTC, Dr. Xiang Liu at Educational Testing Service, Dr. Renjun Hu at Alibaba Cloud, and Dr. Yifei Yuan at University of Copenhagen.
I am seeking a PhD position for the Fall 2025 semester.
News
Oct 15, 2024 | Check out our Awesome LM Evaluation Methodologies, a collection of frontier papers in LM Eval Methodologies! |
---|---|
Sep 27, 2024 | PertEval and CCAT are accepted by NeurIPS 2024. PertEval is selected as a Spotlight paper. |
May 30, 2024 | Finish my research intern at Alibaba Cloud. Many thanks to my mentor and all collaborators! ![]() ![]() |
May 30, 2024 | PertEval is available at Arxiv. [Paper] |
Jan 23, 2024 | ID-CDF is accepted by TheWebConf 2024. [Paper] |
Jan 06, 2024 | Start my research intern in LLM evaluation at Alibaba Cloud! |
Sep 18, 2023 | DeepEval is available at Arxiv. [Paper] |
Mar 18, 2023 | One research about Bayesian Item Response Theory is invited to make an oral presentation at IMPS 2023, University of Maryland, College Park, USA. [Web] |
May 20, 2022 | HierCDF is accepted by SIGKDD 2022. [Paper] |